Imperial College London researchers have created a machine learning method that may identify Alzheimer’s disease from a single magnetic resonance imaging MRI scan by examining structural patterns within the brain, including areas not previously connected with Alzheimer’s. The technique’s advantages, according to the scientists, include its simplicity and the ability to detect the disease at an early stage, when Alzheimer’s can otherwise be difficult to diagnose.
“Currently, no other simple and generally available approaches can predict Alzheimer’s disease with this degree of accuracy,” stated research lead Eric Aboagye, PhD, professor at Imperial’s department of surgery and cancer. Many patients who report with Alzheimer’s at memory clinics also have other neurological disorders, but our system was able to distinguish between those who had Alzheimer’s and those who did not.”
In a publication titled “A prediction model employing the mesoscopic architecture of the living brain to detect Alzheimer’s disease,” Aboagye and colleagues concluded, “This new data analysis method has potential for boosting the accuracy of Alzheimer diagnosis.”
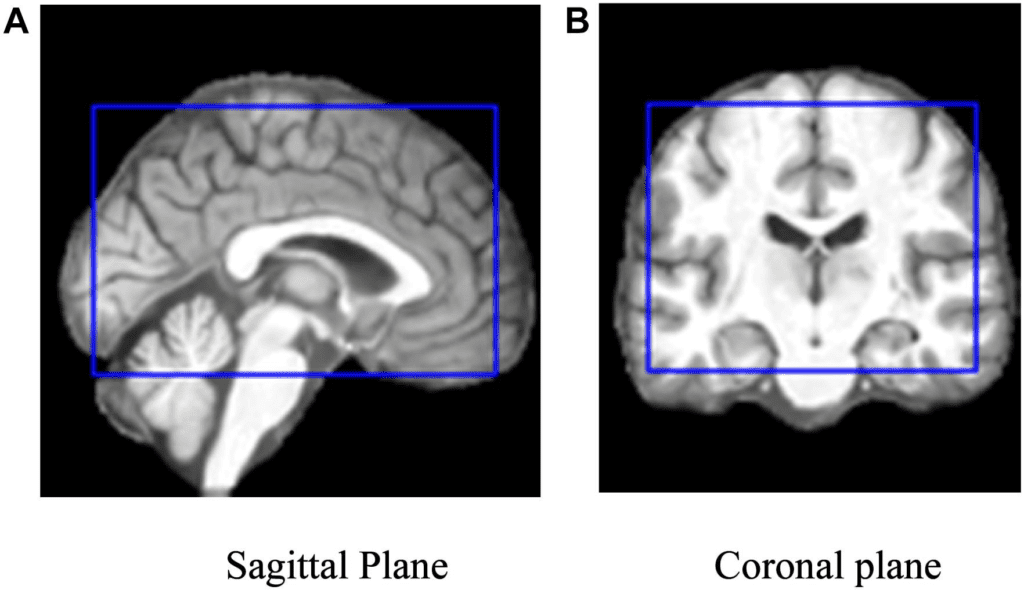
According to the authors, Alzheimer’s disease is the most frequent form of dementia, affecting memory, thinking, and behaviour. In the United Kingdom, the disorder affects approximately 500,000 people, the majority of whom are over the age of 65, while younger people can also develop Alzheimer’s. Memory loss and difficulties with thinking, problem solving, and language are the most common symptoms of dementia.
Although there is no cure for Alzheimer’s disease, people benefit from receiving a diagnosis as soon as possible. It enables them to seek assistance and support, receive therapy to manage their symptoms, and plan for the future. Accurately identifying patients at an early stage of the disease would also assist researchers understand the brain alterations that cause the disease, as well as support the creation and testing of new treatments. “It can be difficult to detect Alzheimer’s disease,” the researchers concluded, “which can lead to suboptimal patient management.”
Alzheimer’s disease is currently diagnosed using a battery of tests, including memory and cognitive exams, as well as brain scans. The scans are used to look for protein deposits in the brain and shrinking of the hippocampus, the memory-related part of the brain. All of these tests can take several weeks to set up and process.
The novel method requires only one MRI scan brain scan performed on a typical 1.5 Tesla equipment, which is found in most hospitals. The researchers applied an algorithm developed for classifying cancer tumours to the brain. They divided the brain into 115 sections and assigned 660 different properties to each region, such as size, shape, and texture. They then trained the algorithm to recognise where changes in these traits may effectively predict the presence of Alzheimer’s disease, even before visible brain shrinkage occurred. “A biomarker named “Alzheimer’s Predictive Vector” (ApV) was developed for each patient using a two-stage least absolute shrinkage and selection operator (LASSO),” the researchers noted.
The team tested their approach on brain scans from over 400 patients with either early- or late-stage Alzheimer’s, healthy controls, and patients with other neurological conditions such as frontotemporal dementia and Parkinson’s disease using data from the Alzheimer’s Disease Neuroimaging Initiative. They also tested the strategy on data from over 80 patients having Alzheimer’s diagnostic testing at Imperial College Healthcare NHS Trust.
They discovered that the MRI-based machine learning system could effectively determine whether a patient had Alzheimer’s disease or not in 98 percent of cases. It could also tell the difference between early and late-stage Alzheimer’s disease in 79 percent of individuals.
“This strategy gives a biomarker capable of detecting an early stage of Alzheimer’s disease with great potential enhancement of the clinical decision support system,” the researchers wrote. “Our ApV is robust and repeatable across MRI images, suggesting its potential for future use in clinical practise.” The method also does not necessitate the involvement of a “subject matter expert,” as it use proven software for both brain segmentation and radiomics analysis, according to the scientists. “The programme computes human engineered features, making the ApV easy to read and enabling clinical translation.”
“Waiting for a diagnosis may be a painful experience for patients and their families,” Aboagye said. It would be really beneficial if we could lessen the length of time patients have to wait, make diagnosis easier, and remove some of the ambiguity. Our novel method could also identify early-stage patients for clinical trials of new pharmacological therapies or lifestyle changes, which is now extremely difficult.”
The novel approach detected alterations in brain areas previously unrelated to Alzheimer’s disease, such as the cerebellum (the part of the brain that organises and regulates physical activity) and the ventral diencephalon (linked to the senses, sight and hearing). This brings up new possibilities for research into these areas and their connections to Alzheimer’s disease.
“Although neuroradiologists already interpret MRI scans to help diagnose Alzheimer’s, there are likely to be features of the scans that aren’t visible, even to specialists,” said co-author Paresh Malhotra, PhD, a consultant neurologist at Imperial College Healthcare NHS Trust and a researcher in Imperial’s department of brain sciences. Using an algorithm that can identify texture and minor structural traits in the brain afflicted by Alzheimer’s disease could greatly improve the information we can acquire from current imaging techniques.”
“… this study proposes an unsupervised method for the establishment of an MRI-based biomarker for the biological characterisation of AD,” the team concluded. The ApV is repeatable and durable. It is quickly computed using manually created features and is ready for integration into the clinical decision support system without the requirement for additional sampling or patient testing.”