A single cell genome or transcriptome can provide much more information about its place in living systems than sequencing a whole batch of cells, just as asking a single person about their health will provide tailored, personalized information impossible to glean from a large poll. However, until recently, the technology to obtain that high resolution genomic data did not exist—and until today, there was no reliable way to ensure the high quality and usefulness of that data.
Dr. Weijun Luo and Dr. Cory Brouwer of the University of North Carolina at Charlotte developed an artificial intelligence algorithm to “clean” noisy single-cell RNA sequencing (scRNA-Seq) data. On April 7, 2022, the study, “A Universal Deep Neural Network for In-Depth Cleaning of Single-Cell RNA-Seq Data,” was published in Nature Communications.
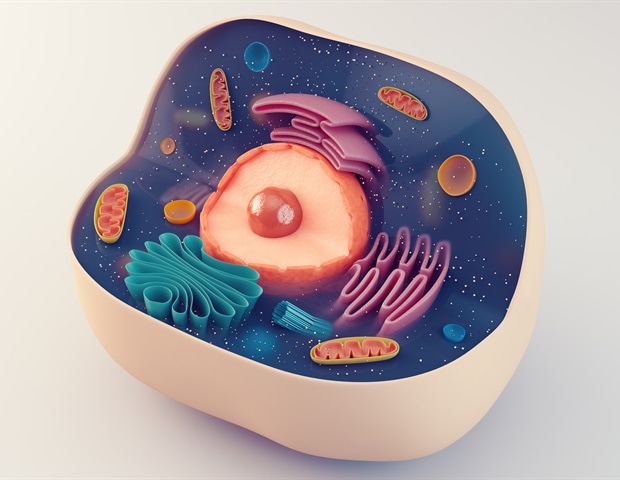
Since the Human Genome Project in the 1990s, scientists have been searching genomes to unlock the secrets of life, from identifying specific genes associated with sickle cell anaemia and breast cancer to developing mRNA vaccines in the ongoing COVID-19 pandemic. Technology has advanced since the days of batching thousands of cells together to decrypt the millions of base pairs that make up genetic information, and in 2009 researchers developed scRNA-Seq, which only sequences the transcriptome or the expressed portion of the genome in a single cell of a living organism and is now widely used in biomedical research.
Unfortunately, scRNA-Seq data is extremely noisy, with numerous errors and quality issues. When a single cell is sequenced rather than many cells, there are many “dropouts”—missing genes in the data. A single cell, like a single person, may have its own health issues or be at an inconvenient stage in its life cycle—it may have just divided or be on its way to cell death—which can cause more errors or technical variations in the scRNA-Seq data. Aside from single-cell-specific issues, genomic profiling is frequently fraught with “normal” sequencing errors. All of these errors must be “cleaned” from the data before it can be used or interpreted, which is where the new AI algorithm enters the picture.

Dr. Luo and his colleagues demonstrated in the study that AutoClass can reconstruct high-quality scRNA-Seq data and improve downstream analysis in a variety of ways. Furthermore, AutoClass is robust and performs well in a wide range of scRNA-Seq data types and conditions.
AutoClass is highly efficient and scalable, and it works well with data of varying sample sizes and feature sizes. It also runs smoothly on a standard PC or laptop. AutoClass is available as open source software online at: